- The Prompt Innovator
- Pages
- Another AI Doomsday Outlook - Unchecked Power Consumption Threatens Climate
Another AI Doomsday Outlook

The Growing Energy Footprint of Artificial Intelligence
As artificial intelligence (AI) continues to evolve and integrate into various aspects of our lives, its demand for energy has soared to unprecedented levels, posing significant challenges for global energy systems and sustainability goals.
Unprecedented Energy Consumption
AI technologies, especially large-scale models like ChatGPT, require massive amounts of energy to function. Data centers, which house these AI systems, are major energy consumers. In 2022, these centers accounted for about 4% of the US's overall energy use. This figure is expected to grow as AI technologies become more widespread【source】. The International Energy Agency (IEA) projects that the global electricity consumption by AI could reach between 620 and 1,050 TWh by 2026, a range equivalent to the energy consumption of entire countries like Sweden or Germany.
Impact on Energy Infrastructure
The surge in AI-driven energy demand is straining the existing power grids and has even led to the postponement of decommissioning coal-fired power plants. For instance, in the Kansas City area, the construction of a data center and a factory for electric-vehicle batteries requires so much energy that local energy providers have had to delay plans for shutting down a coal plant. This reflects a broader trend across the United States, where electricity consumption at data centers alone is expected to triple by the end of the decade.
Sustainability Challenges
The high energy demands of AI are increasingly at odds with the climate action goals of many tech companies, which have made commitments to reduce their carbon emissions and achieve net-zero targets. However, the reality is that many are turning to fossil fuels like natural gas to meet their energy needs. This shift poses a significant challenge to their sustainability pledges and the broader goals of reducing greenhouse gas emissions.
The carbon emissions from AI use are influenced by several factors, including the energy sources powering the data centers, the efficiency of the AI models, and the operational practices of the data centers. In 2020, it was found that training a model like GPT-3 could emit 502 metric tons of CO2, equivalent to driving 112 gasoline-powered cars for a year (Source: Columbia Climate School). The operations for AI models, especially during inference processes, can be very energy-intensive, consuming even more energy than the training phase
This might be your grand childrens childhood dream of playing in the forest:
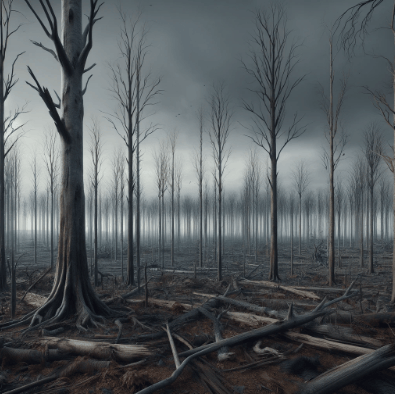
Here's a more detailed look at AI's electricity use and its related climate issues:
- Electricity Consumption: AI and data center operations are significant electricity consumers. For example, training a model like GPT-3 can consume 1287 MWh of electricity, with subsequent carbon emissions comparable to annual emissions from over a hundred gasoline-powered cars.
- Regional Variations in Emissions: The carbon footprint of AI operations varies widely by region, largely dependent on the local energy mix. AI operations in areas with greener grids (like France) have significantly lower emissions compared to those in areas reliant on fossil fuels【source】.
- Inference vs. Training Energy Use: While training AI models is energy-intensive, the inference phase (when AI models are actively used to make predictions) can consume even more energy. In some estimates, inference accounts for about 60% of the total energy used by AI systems.
- Rapid Increase in Workloads: The workloads managed by large data centers have been growing rapidly, at about 20-40% annually, leading to a substantial increase in electricity use. For example, combined electricity usage by major tech firms more than doubled from 2017 to 2021.
- Efficiency Improvements and Jevons Paradox: Despite efficiency gains in AI operations, increased efficiency can lead to greater overall consumption—a phenomenon known as the Jevons paradox. This paradox highlights that as AI becomes more energy efficient, its applications expand, potentially leading to increased overall energy consumption.
- Water Use for Cooling: Data centers use substantial amounts of water for cooling, which also raises environmental concerns, especially in water-scarce regions. In 2022, Google's data centers were reported to consume billions of gallons of water, intensifying local concerns about water resource competition.
- Policies for Transparency and Reduction: Increasing transparency regarding the carbon and environmental footprint of AI operations is seen as crucial for managing and potentially reducing their impact. This includes requirements for detailed reporting on energy use and emissions from AI operations【source】.
- Shift Towards Renewable Energy: Major cloud service providers are moving towards renewable energy sources to power their operations. Companies like Microsoft and Google have set ambitious targets to run their data centers on 100% renewable energy within the next decade